- Library
- Podcasts
- Generationship
- Ep. #24, Nudge with Jacqueline-Amadea Pely and Desiree-Jessica Pely, PhD
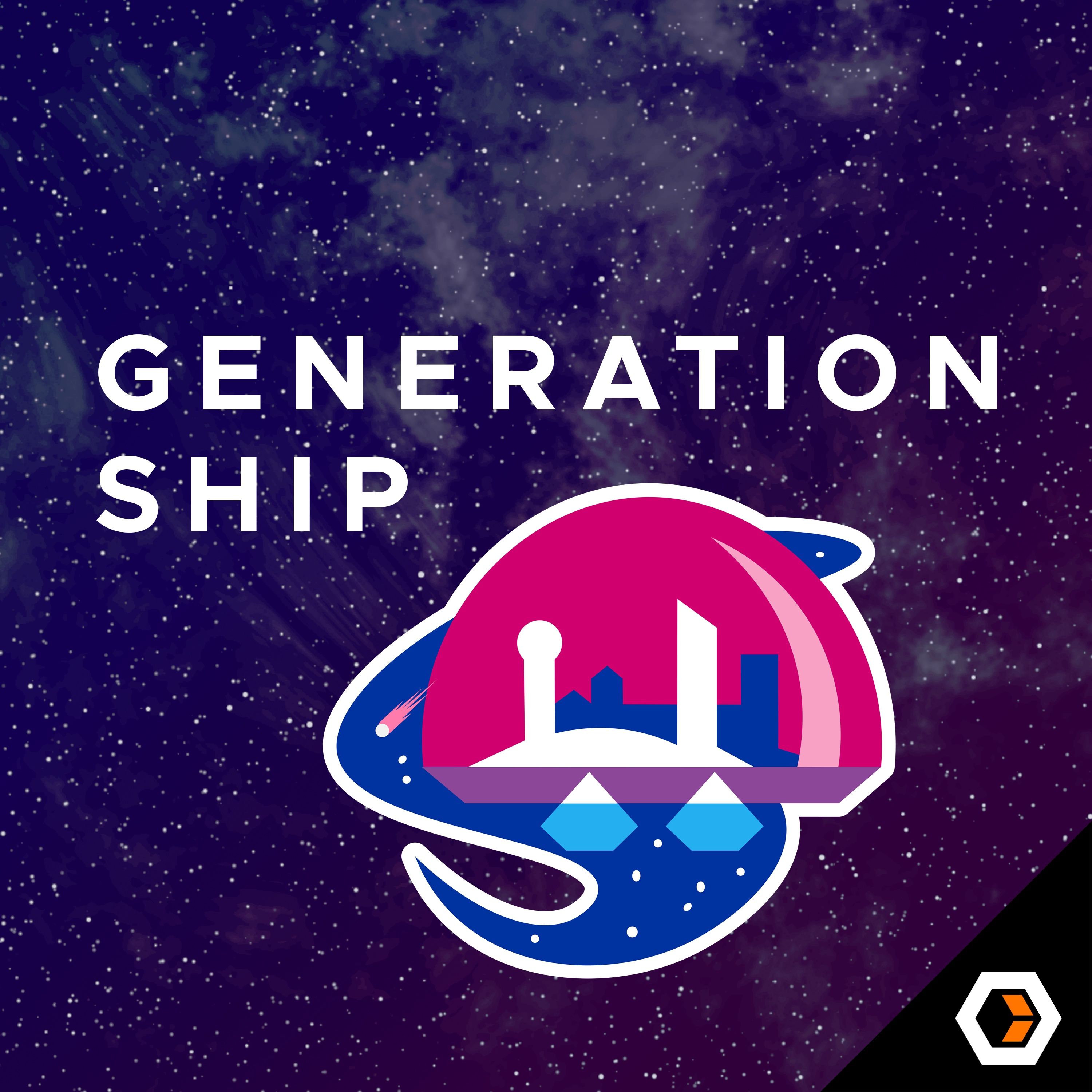
Ep. #24, Nudge with Jacqueline-Amadea Pely and Desiree-Jessica Pely, PhD
In episode 24 of Generationship, Rachel Chalmers speaks with Dr. Desiree-Jessica Pely and Jacqueline-Amadea Pely, co-founders of LoyeeAi. The sisters share insights on how AI and behavioral economics can be used to improve decision-making in sales and beyond, while discussing the potential benefits and risks of large language models. Tune in for a thoughtful conversation on the future of AI, human collaboration, and responsible innovation.
Jacqueline-Amadea Pely is the co-founder and Chief Product Officer of LoyeeAi, where she focuses on leveraging AI to improve sales processes by aligning buyers with the right products. With a background in Media, Communications, and Technology Management, Ama has played a key role in building innovative AI-driven tools that enhance decision-making and foster productivity in sales teams.
Dr. Desiree-Jessica Pely is the co-founder and CEO of LoyeeAi, an AI SaaS platform that utilizes behavioral economics to optimize sales strategies. She holds a PhD in Financial and Behavioral Economics and studied under Nobel Prize-winning economist Richard Thaler, applying her expertise to develop AI tools that support human decision-making.
transcript
Rachel Chalmers: Today, I am so delighted to welcome Dr. Desiree-Jessica Pely and Jacqueline-Amadea Pely to the show.
Jess is currently the founder and CEO of AI company, Loyee.io which is her second startup. She has a PhD in financial and behavioral economics from the University of Munich and has studied at Yale School of Management.
Ama is founder and CPO of Loyee, which is also her second time as a founder. She started her entrepreneurship journey directly after her master's in science from UC Berkeley and the University of Munich.
Jess, Ama, welcome to this show. It's wonderful to have you.
Dr. Desiree-Jessica "Jess" Pely: Thanks for having us.
Rachel: You are proteges of Richard Thaler, the Nobel Prize winner for economics. Jess, can you maybe talk a little bit about what you learned from him?
Jess: Yes, of course. So I did my PhD in financial economics, and obviously, I did a lot of research in that field and I had, you know, had the luck to work with Richard Thaler, and Richard Thaler is known for his contributions to behavioral economics.
So it basically summarizes how us humans make maybe decisions that are not always in our best interest or not always very rational. And it really taught me and influenced also how we built our products, how we think about humans making decisions on a daily basis, you know?
As people, we are not always acting rationally. You know, we might be sometimes lazy, might have limited cognitive abilities or just like follow inconsistent preferences, and this is on a daily basis. Decisions are very complex in nowadays world, and you know, Richard Thaler taught us how we can design products, solutions, provide incentives to improve decisions that the society is making.
So for example, we could ethically nudge humans by automating certain workflows to make better and faster decisions.
Rachel: People may know Thaler's work from his book, "Nudge," which popularized a lot of what he had been doing, and I love the canonical example of making a 401(k) election opt-out rather than opt-in, which greatly improves retention and participation in 401(k) plans.
It's just a way of using our human biases to optimize for the outcomes that we want, rather than leaving things to slightly more chance and ape brain.
I find it a really rich field as we're moving into this age of large language models because to me, the excitement of this new generation of AI is partnering with machines and having humans be amplified or augmented by them and designing those interactions for good outcomes.
Is that something that you think about as you work on what you're building for Loyee?
Jess: Yeah, I love also the 401(k) example because yeah, it really shows us that nudges can live forever.
That was actually the paper I was supporting with, and this is obviously the groundwork also for our company, Loyee, because while I was doing my PhD, I was researching obviously how, you know, behavioral biases might prevent certain decisions and it's really instrumental on how we build products, how we build those machines, those AI machines that we work with nowadays almost on a daily basis.
So yeah, to give you an example from Loyee, is for example, we help sales teams and go to market teams make right decisions when finding and targeting their prospects and also support prospects or potential buyers to buy software products to find the best fitting products, because we need to build a recommendation system of what is good and what is not good, and obviously machines, AI, technology can help us with that, but it still needs someone like us, at least at this stage, that need to design this product and build this product that it can be used.
So we have the responsibility, what will spit out, even though we are using AI in those machines.
Rachel: Yeah, absolutely. It doesn't replace human ingenuity. It ideally supports it and makes it more powerful. Can you give us a sense of what people are using Loyee for? What are some of your coolest customer case studies?
Jacqueline-Amadea "Ama" Pely: I can jump in here, so in general, we are in the sales space or go to market space so we help sales reps to automate that part that they currently do manually and don't like.
The problem that we see is that mass outreach, reaching out to all the companies out there and trying to hope for an answer that somebody just tells you back, "Hey, I want to connect to you, I want to see what your product does," it's just not working anymore, so there must be more of a quality aspect there where AI can help.
So we come here into place and look into, what are the companies that actually need my product? So it's not about quantity and reaching out to everybody, but more about quality, like, who is having the buying signals that they need the software, and that's where we leverage AI.
We go through publicly available data but also through other sources that are on, for example, what kind of technologies does the company use and so on to map out what do they need, and so the rep knows exactly who to target and this company apparently will have a higher interest to get in touch.
Jess: Yeah I think this is, like what we are building, there is a great example of how AI can help because what we know is that everybody hates to be cold outreached.
Like, I hate, every morning when I wake up having like millions of emails, just kidding, like hundreds of emails in my inbox that are written by AI bots, it really is not fun.
And now, you know, back in the days, it wasn't fun, but now it's just like increased, so we have to build now even better technology and better products that really distinguishes what is worth it, what is relevant to me, what is timely to me, versus just shooting out messages.
And this is exactly where Loyee comes into place, that we don't want to automate messaging but we want to provide higher quality and really match the best products to the best potential buyers.
And in terms of our mission with the companies, to really enhance human productivity and wellbeing, which is also ingrained in our name, Loyee, so it's a wordplay between like employees and loyalty, and this is always very important to us that, you know, we don't become this product that annoys people but that really enables people.
Rachel: Yeah, because as much as we all hate getting blind emails and cold outreach, how great is it when somebody draws your attention to exactly the product you need at the moment that you need it?
That's a fantastic feeling when you're like, "Oh yes, "I had been looking for something like this "without noticing it." And if we can re-engineer sales outreach to have more of those exciting moments, then it's great for everyone.
Jess: Exactly, and you know, like talking about making decisions, right, like, sales reps oftentimes don't know who to go after and why, so we need to help them, guide them in the decision to figuring this out.
But also on the buying side, 50% of software purchases are a waste that people regretted, so we really have to educate also the buyers, what their pains are and how they can solve for certain problems and to make better decision on that end, so we can really nudge them with good quality insights leveraging AI.
Rachel: Ama back to you, are you worried at all about the risks of the widespread use of commercial LLMs? Are we baking algorithmic bias into our systems?
Ama: I'm more on the positive side. I think a lot of things will be automated that anyways human hate to do. Like for example, a good topic that we're talking about, manual research.
Like, nobody really likes to do it and if a system can do it for me, I'm very happy because now I have time to do actually the things that are more fun that involve human interaction and things that are not going to be solved with AI.
And I also think there is lots of potential in solving problems that our brain is just not capable of doing with going through large amounts of data and solving problems in healthcare or climate that are just not solvable these days, so I see it more on the positive side.
Obviously, I think there need to be regulations in place to avoid certain problems, but I think, you know, when those regulations will be there, there is just a really positive side to it. That's my biased opinion, but we will all figure that out in the next years.
Rachel: Jess, how do you think about risk mitigation, using these sort of black box language models?
Jess: I think a lot of it starts with education, because I do believe that, yeah, you know, there is more to come and especially AI and LLMs are definitely, you know, in their, how do you say, in their baby steps. I think there is a lot to come, but we really need to educate.
Because what I also noticed with our customers is they always have very high expectations of what models do for them, and it's almost like they expect perfection from models to a higher degree than from humans, even though we know that humans make decisions, why would an AI make less decisions?
And I think it starts with education. Like, we have to educate our customers that the AI that we are using, that we developed, that we programmed is an intern, and you have to be open to guide the intern, to educate the intern, and to also teach the intern, and oftentimes, there is not the understanding on the customer side that it's just an intern that we have to enable and we have to correct for this lack of information.
Obviously in the future, it will be better and maybe better than just an intern, we all hope and expect that, but to mitigate for that risk is really educating the customers and also using different models for quality checks.
So for example, our product has one principle that we always want to provide the best quality, but we also leverage different models to check for this quality. So you know, to prevent from biases, we can ask different models, "Okay, might this be a biased answer?"
And then really correct also with AI and large language models for our-- Correct the models in an automated way, so this is something that we do on our end, but I am sure that having like a system in place or like principles in place to account for it at least, and then, you know, having someone who's responsible for it, like us in this case, the founders, is very important.
Rachel: Yeah, I think it's great that there are founders in the field who are as thoughtful as you. I do get worried when people dismiss the bias and the risks. I think it's more important to address those head on.
And on a related note, you know, you've been working in machine learning for years and now language models come along and there's this enormous hype wave and the valuation's going through the stratosphere.
Do you worry that the claims for this technology are overblown? What happens when there's a wave of disillusionment?
Jess: Yeah, good question. So maybe to summarize it, so I did a lot of NLP research and used machine learning models during my PhD.
All this is by now super outdated, so now, we are not developing our own LLM models but we really leverage what is out there. So, and this is of tremendous help because it's just so much better than what I did a couple of years ago in my PhD.
Is there a worry on the gen AI hype? I think I'm on Ama's page, that I'm rather optimistic than pessimistic about it because I love the fact that it drives innovation and we need innovation.
I'm just more worried about that people don't know how much investment it still takes to make something good. You know, starting with educating the buyers or the users of these technologies, and really also understanding the error rates of it.
You know, there are studies that show if a human does a data collection, the error rate for simple task is 10%, for average task 20%. Why do we expect perfection from AI? I think this is something where we really have to dive deeper.
You know, what keeps me up at night is more that new biases will arise and we might not even know about these biases and it takes maybe a research group or people that are at the forefront of technology that really understand those concepts and consequences that might come along with it.
Rachel: That totally makes sense. With so much research being published all the time, how do the two of you stay current? What are some of your favorite sources for learning about AI?
Ama: I just read a fun paper that said, you know, the nicer you are to a gen AI LLM model, the better results it gets you.
So it's kind of interesting to follow, like the recent publications there because you get also insight into, you know, how to better prompt LLMs and so on. I know Jess, you like to go into webinars as well and, you know, following the recent news of the large language models that we all use as tools, like for example, from OpenAI, but feel free to share also here, Jess.
Jess: I mean, I'm an academic, so I always go to the, you know, schools that have online classes, you know, from Stanford or Harvard. There are some cool courses that you can actually take, so I really love those.
You know, more practically, I love to listen to podcasts, even podcasts like this one. I find it always interesting when you have like different speakers, you know, and they provide like their thoughts on it. Obviously it's one opinion and one perspective, but I think it still is a good food for thought.
And in terms of, you know, for our own product, yeah, all the updates also from OpenAI and so on, it's really cool to see how they develop, how they think about their product, what their product roadmap is, so that's probably the sources that I look into.
Rachel: Very cool. Ama, I also love that paper about, you know, be kind to your language model, because a prompt is a nudge and the way we design prompts influences the kinds of behavior we can expect to see out of the models. It's fascinating.
Ama: Yeah, sometimes I feel silly, though when I really think about it. I'm like, is this real? I'm just talking to a computer, but it's, yeah, it's funny.
Rachel: I think in some ways, these AIs are like children, or interns, and you have to raise them and you raise them in the culture that you want them to propagate and put out into the world.
Jess: That's a nice way of thinking. That's actually the right mindset we should all have because then it would also prevent, you know, the language models to have those biases.
Rachel: It would be great to think so. I'm going to make you both empresses of the galaxy. Everything goes the way you would like it to for the next five years. What does the future look like?
Ama: Well, I think with AI, I think what would be really nice is when more people or many people actually understand AI.
Because most of us actually just use it as a tool, but nobody really understands the technology behind it and it's highly complex and everything, but I think understanding in a broader sense would be really, really good to avoid human biases and go into the right direction and avoid things that we are scared of with AI. And I hope we use those models, really, to solve issues that are currently not solvable in areas that really impact the world and that we leverage it to the full extent because I think there is lots of potential.
Jess: Yeah, like in the next five years, I'm very optimistic that we will, you know, use AI to help us make better decisions, but also to stay educated, because the worry that I have is that when you have these amazing tools is that, you know, humankind stops thinking and kind of delegating everything, so I think my wish would be is to have, really, a big partner by your side that helps you make decisions, not as decisions for you, and that as humans, still remain responsible and accountable for our decisions.
I think this is something that is very important, and you know, especially in the knowledge workers, you know, all these tasks that are really redundant sometimes that really get automated to a degree that we have space, where humankind can really focus on the big issues that we are having nowadays, that would make me happy.
Rachel: That's a great vision. Finally, my favorite question, you know, we call the podcast Generationship because we're optimistic, we believe like, humans are on a spaceship traveling into the future, we've got to learn to share our resources.
If you were on a generation ship journeying to the stars, what would you name that ship?
Ama: I would name it Explore Express. Because I believe in never stopping exploring, you know, in every direction. Like, never stop exploring, never stop learning about new things, and I think it's very important to have this mindset.
And being on a starship would mean going somewhere where you don't really know what waits there for you and being open-minded and open to exploring. That's why I would call it Explore Express.
Rachel: That's great. Well, and the universe is so interesting. Jess?
Jess: Yeah, I was thinking about it. It's really tough to make a decision here, but probably something around Elysium Odyssey.
So you know, the concept of Elysium is like really a place of ideal happiness and it comes from like the ancient Greeks, but like, having also the idea of an epic journey, the odyssey, is, you know, we want this adventure, we want to make this adventurous expedition to create something new, like a new utopia among all the stars that are out there.
So yeah, I think that's what I envision.
Rachel: Explore Express and Elysium Odyssey, they're both beautiful names.
Thank you both so much for coming on the show. Good luck with everything. It's been a delight to chat.
Ama: Thank you for having us.
Content from the Library
Platform Builders Ep. #9, Manny Medina Explains: What the F Is an AI Agent?
In episode 9 of Platform Builders, Christine and Isaac sit down with Manny Medina, founder and CEO of Paid, to unpack the...
LLM Fine-Tuning: A Guide for Engineering Teams in 2025
General-purpose large language models (LLMs) are built for broad artificial intelligence (AI) applications. The most popular...
Platform Builders Ep. #8, The Beauty of Vertical SaaS with John Melas-Kyriazi of Standard Metrics
In episode 8 of Platform Builders, Christine Spang and Isaac Nassimi are joined by John Melas-Kyriazi, founder and CEO of...